Guidelines for Data WareHouse (DWH) Implementation
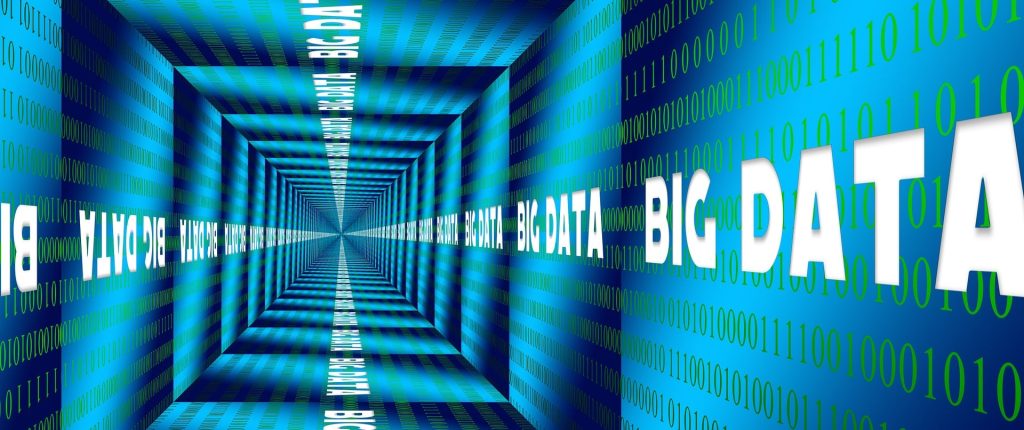
A Data Warehouse (DWH) is a data storage facility for dispositive data.
This data is topic-oriented and logically centralized. A DWH integrates business’ huge datasets from various operative internal systems a well as external systems.
Ideally, it serves as a company-wide, uniform, and consistent database for all types of decision support systems.
The data warehouse is usually a core component of comprehensive business intelligence (BI) concepts.
Implementation of Data WareHouse
Data warehousing can be defined as the use of a data warehouse in a concrete application environment – an analysis environment.
There are basically four approaches to implementing a data warehouse – classic, real-time, closed-loop, and active data warehousing.
- Classical Data Warehousing
This allows read-only access to the warehouse where the data will be updated periodically during analysis.
This type of data supply is the main form of data connection and is primarily employed in standardized planning and control systems with a short to medium-term decision horizon.
- Closed-loop Data warehousing
This approach enables the analysis results to be fed back into the operative and other dispositive data sets.
Therefore, more/updated information will be added to the datasets, which will be useful in the implementation of decisions or for further analysis.
For example, in Customer Relationship Management, the insights from customer segments, on the basis of BI analyses can be integrated into the operational data.
This serves as an additional or structural data that will assist in automated campaign execution with these customers (target groups).
- Real-time Data Warehousing
This approach refers to the direct, transaction-related data supply of the WareHouse so that analysis can be based on real-time data.
This is very important in an industry where emergency decisions that are very sensitive to time can be executed.
For example, stock exchanges and security trading where analysis can be void with respect to changes in time.
- Active Data Warehousing
This is also based on closed-loop data warehousing. It adds operational components to the analyses by triggering automated actions based on specific data constellations.
In this way, structured, recurring decision-making can be executed effectively. For example, when solving logistical optimization problems in the delivery and freight area.
Conclusion
The aforementioned implementation approaches can help businesses make an effective decision on various operational problems.
Both Classical, Closed-loop, Realtime, and Active Data Warehousing make a complete traditional solution.
Several data warehousing approaches are usually implemented in parallel in powerful Data Warehouse concepts.
The concrete design of these approaches must always be tailored to the individual organization and based on the real requirements of the company.