Meaning of Big Data Analysis and Uses
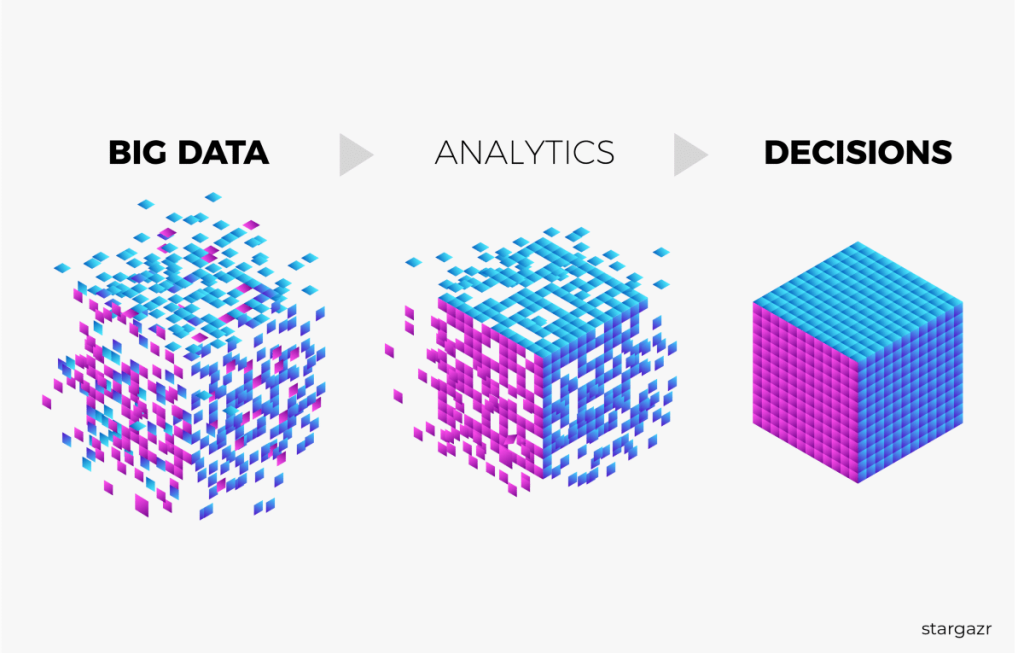
Big Data Analysis Meaning
Data analysis deals with the questioning and exploration of data sets using various quantitative methods with the help of big data analytics tools.
Big data analysis is partly motivated by statistical modeling or machine learning.
In contrast to Business Intelligence, data analysis extends beyond descriptive analysis and often has a predictive component.
The method to be used depends on the concrete business case as well as on data quality and availability.
How Big Data’s Analysis is carried out
The starting point for each analysis project is different. In order to estimate the potential of data analysis, it is necessary to examine the four core areas – business needs, data, data analysis, and (IT) infrastructure:
Business needs
The accuracy of the problem description and scope varies from case to case.
In some cases the guiding questions and scopes that guide the analysis phase are formulated very precisely, in other cases they must be worked out and refined during the process.
Data
The data to be used in the project may already have been defined or a suitable source is not yet clear.
The scope and quality of the data essentially determine the progress of the further process.
Parameters are, for example, the structure (i.e. the degree of processing) or the size of the data set (is it a CSV file or a complex large database).
Analysis
Which methods are used varies from case to case and must be tested and investigated?
In most cases, an initial data exploration will be the first step before the granularity of the questions underlying the analysis increases.
Infrastructure
The current (technical) status of the department (e.g. own data warehouse, reporting system) or own resources and competencies are other important aspects.
Data Analysis Methods
Methods from various disciplines such as statistics, economics, or computer science are applied to identify patterns, influencing factors, or dependencies.
Improved analysis of internal data. One example is forecasting methods that supplement expert-based planning approaches with additional key figures.
These methods build on existing databases such as business intelligence systems and incorporate new or additional findings into internal company processes.
New combinations of data sets offer new insights, for example by combining sensor data and user profiles.
Tapping new or (previously) unused data sources (e.g. websites, open data) to identify the potential for generating new insights. However, a context or a predefined application is required to use the data.
An example in this context is social media data that can be used for market observation.
However, the core problem in data analysis is to develop the right guiding questions.
In this context, it is important to achieve a match between business needs, potential data sources, and analysis procedures.